Blog
Replicating Experimental Findings with Online Panels
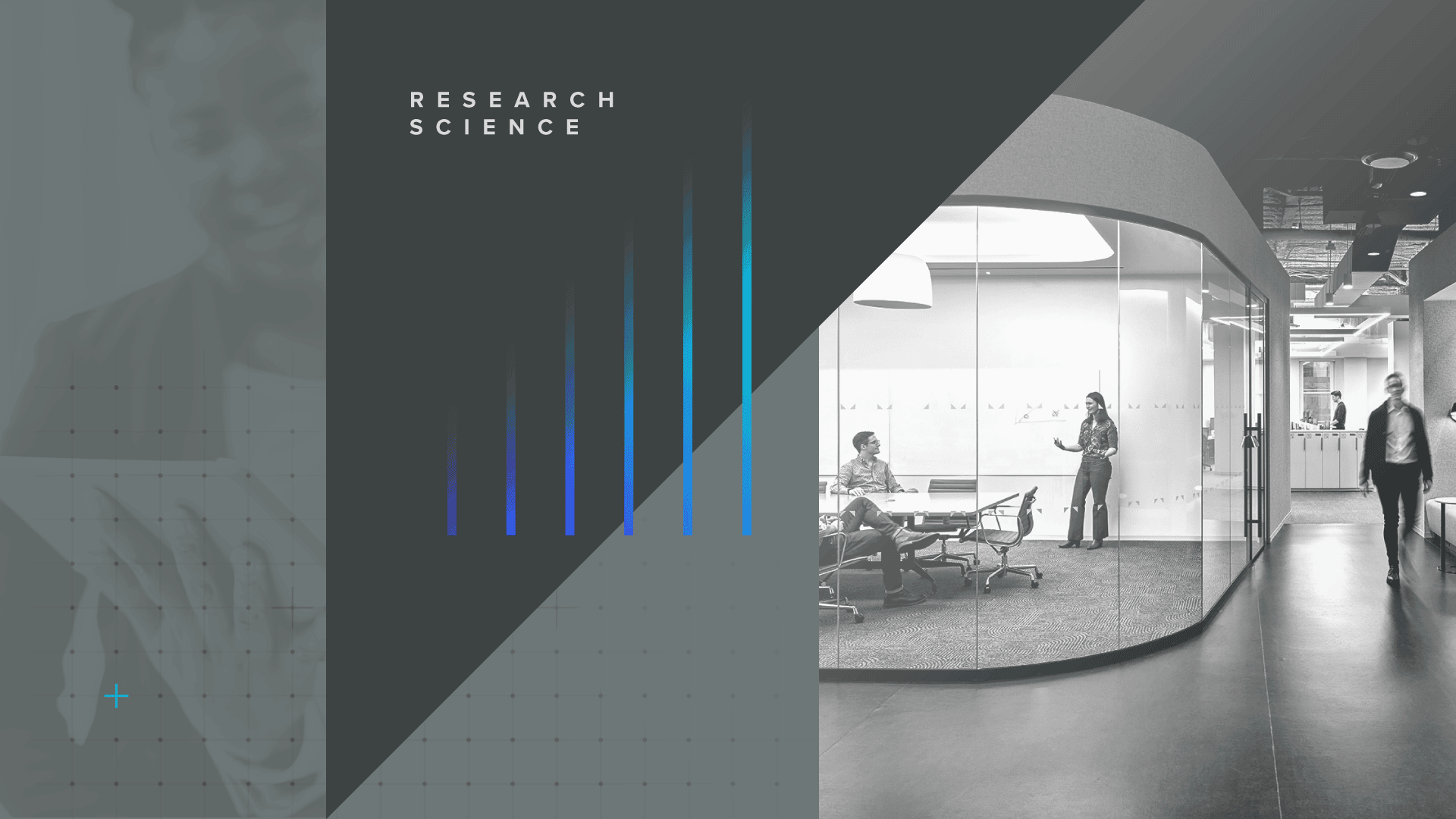
Note: This “How We Do it” post summarizes research shared at the AAPOR 79th Annual Conference in Atlanta, GA presented in a paper session titled “Are You Paying Attention: The Role of Attention Checks in Online Surveys.”
Over the last two decades, some scholars have argued that there is a “replication crisis” in various fields like psychology (Open Science Collaboration 2015), medicine (Ioannidis 2005), and economics (Maniadis et al. 2017). Specifically, several landmark papers have shown that well-known studies often have smaller effect sizes when replicated, and in some cases replication studies have even found effects in the opposite direction (e.g., Bargh et al. 1996 and Doyen et al. 2012).
Although there are many different explanations for these replication failures, some have suggested that the increasing use of online non-probability samples in the social sciences is contributing to these issues (Buzbas et al. 2023, Jerit and Barabas 2023).
At the same time, recent research has noted a decline in the quality of survey data collected online (Aranow et al. 2020; Mercer and Lau, 2023). Various streams of research have identified high rates of misrepresentation in online studies (Agan et al., 2023), suspected fraudulent respondents from well known panels (Bell and Gift, 2024), and inattentive respondents that bias survey estimates (Mercer et al. 2024). Some would argue that poor quality respondents are so prevalent on these panels that we can no longer expect experimental results to replicate when using them.
Given these assertions about online non-probability samples, we conducted a large, nationally representative survey on three separate non-probability panels that included a framing experiment, an information experiment, and a conjoint experiment. We then used the data to replicate the results published in peer-reviewed journals.
We find that off-the-shelf sample from all three panels was able to replicate the direction of results for all three experiments, but that effect sizes were somewhat smaller than the published results. However, when low-quality responses were removed from the three samples using Morning Consult’s standard practices of effective attention checks, tech-enabled respondent deduplication, and fraud detection, effect sizes were significantly larger. In other words, we find that proper data hygiene allows researchers to use online, non-probability samples to produce high-quality, replicable results.
Our Study
To explore whether our framework for identifying high-quality responses allows us to use online non-probability panels to replicate well-known survey results, we conducted a study that included nationally representative samples of US adults from three different non-probability sources,¹ collecting 2,000 responses from each source between March 25th and March 30th 2024. We weighted each of the three samples to population targets of gender, age, education, race, ethnicity, and geographic region, as well as several joint distributions of those variables.
The survey included several attention checks as well as our solutions for identifying duplicate responses and survey fraud. We recorded information about whether each respondent failed each quality check, but all respondents completed the full survey regardless of their performance on these checks. This allows us to compare the full, “off-the-shelf” sample to the more limited set of responses that would have passed all of our quality control measures.²
Finally, the survey included three different experiments and other content. The experiments we selected are well-known and have been replicated in the academic literature.
Our overall analytical approach is to compare average treatment effects among the full set of respondents to average treatment effects among the set of “good” respondents. The theory here is simple — if respondents are not paying attention to experimental treatments, the treatment effect should be biased towards zero. We also compare the average treatment effects from our survey to external benchmarks from peer-reviewed research articles. These benchmarks allow us to evaluate whether non-probability samples can replicate the direction and magnitude of effects.
Experiment 1: Tversky and Kanheman’s Unusual Disease Experiment
Our first experiment was a classic framing experiment — Tversky and Kanheman’s unusual disease experiment. In the experiment, respondents are asked to choose between two programs to try to save people from a disease outbreak. One treatment presents the options in terms of lives saved and the other in terms of lives lost, but the expected value of deaths of each program are exactly the same.
Imagine that your country is preparing for the outbreak of an unusual disease, which is expected to kill 600 people. Two alternative programs to combat the disease have been proposed. Assume that the exact scientific estimates of the consequences of the programs are as follows:
[Lives Saved Frame]
If Program A is adopted, 200 people will be saved. If Program B is adopted, there is 1/3 probability that 600 people will be saved, and 2/3 probability that no people will be saved.
[Lives Lost Frame]
If Program A is adopted, 400 people will die. If Program B is adopted there is 1/3 probability that nobody will die, and 2/3 probability that 600 people will die.
Which of the two programs would you favor?
Tversky and Kahneman find that when framed in terms of lives saved, respondents tend to choose the risk-averse program, but when framed in terms of lives lost, respondents tend to choose the risk-seeking program. The same result has been replicated dozens of times.
We expect that if respondents are not paying attention — if they’re not actually reading the text of the experiment — they should be equally likely to select either program, and our average treatment effect will be biased towards zero.
Figure 1 presents the average treatment effect among all respondents and among the subset of good respondents. The vertical dark gray bar represents the ATE estimated in a recent replication of the disease framing experiment.
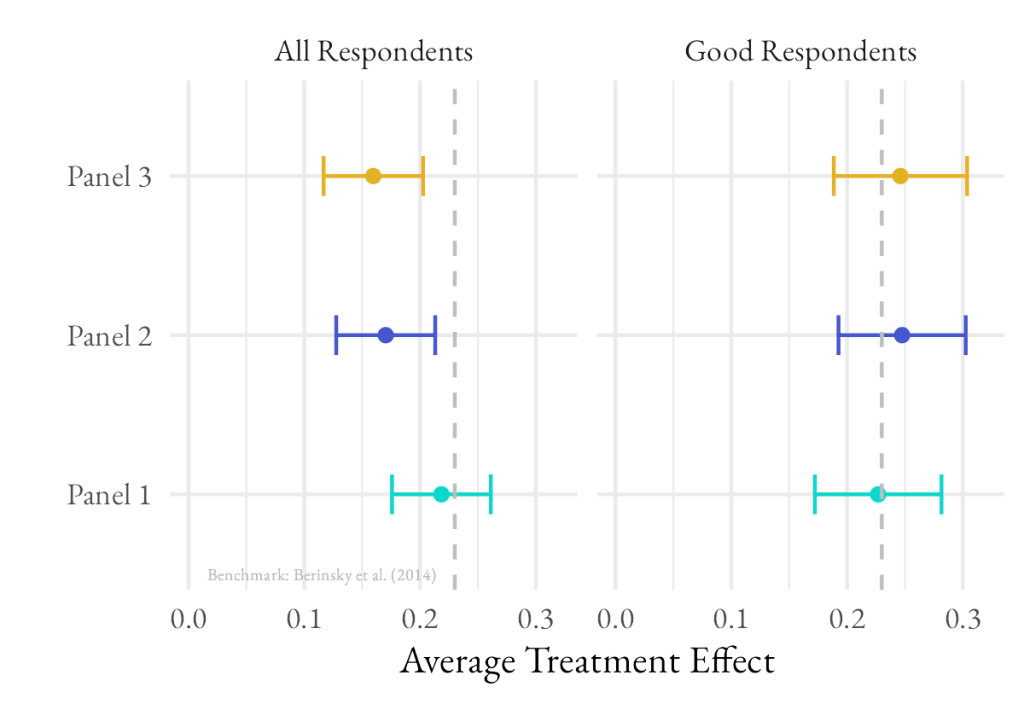
Among the full set of respondents in all three panels, we see a statistically significant treatment effect in the same direction as the Berinsky et al. replication. On average, the effect size among all respondents was around 17% lower than the original replication. After removing duplicate, inattentive, and fraudulent respondents, effect sizes were 25% larger, with the difference between the average treatment effects for all three panels and the Berinsky replication falling within the standard error.
Experiment 2: Piston’s Estate Tax Information Experiment
Next, we replicated Piston’s (2018) Estate Tax experiment. This experiment asks respondents whether they favor or oppose the federal estate tax on inheritances. The treatment group is given additional information about who is affected by the Estate Tax:
[Control Group]
Do you favor or oppose the federal estate tax on inheritances?
[Treatment Group]
As you may know, the federal estate tax on inheritances applies to those who have over $11.18 million, which makes up the wealthiest 0.0006% of Americans. Do you favor or oppose the federal estate tax on inheritances?
Piston finds that the additional information about who pays estate taxes tends to increase support for the Estate Tax. Others have replicated this finding with very similar results.
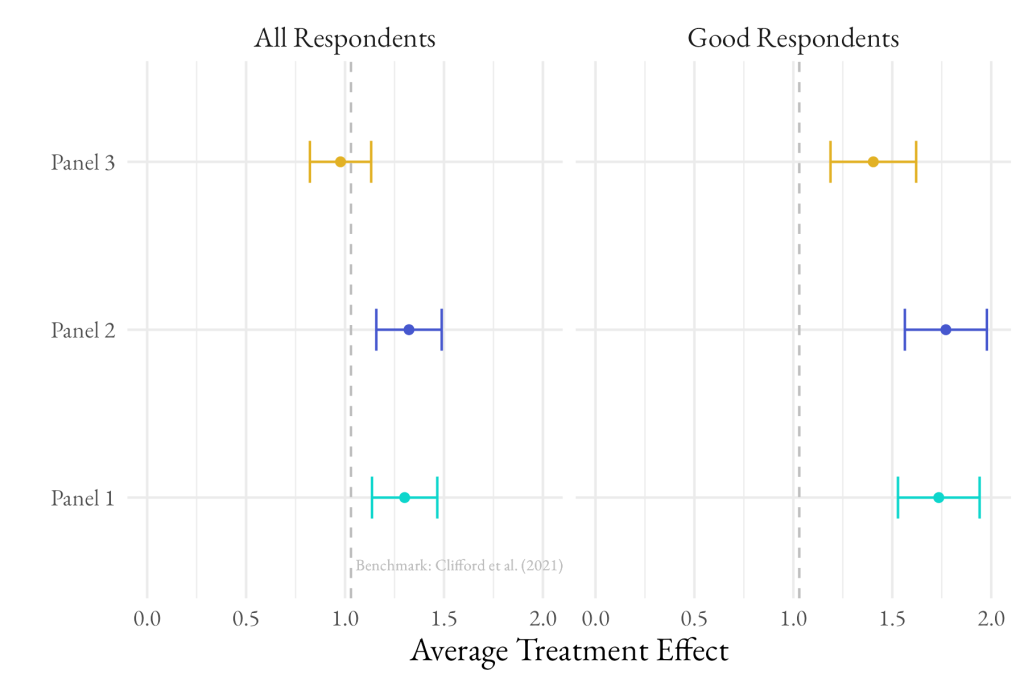
Similar to experiment one, we see a statistically significant effect in the same direction as the original paper among the full set of respondents in each of the three panels. After removing low-quality responses, the treatment effect is 30 to 43% larger. It is worth noting that the benchmark survey from Clifford et al. (2021) also used an online non-probability panel. It is possible that had the authors had better tools for removing poor quality respondents, their effect sizes might have been significantly larger than reported.
Experiment 3: Hainmueller and Hopkins’ Immigration Conjoint
Finally, we included a conjoint experiment designed by Hainmueller and Hopkins (2015) to measure attitudes towards immigrants. In the experiment, respondents are shown two randomized profiles of hypothetical immigrants. Respondents are instructed to act as if they were an immigration official and asked to choose which of the two immigrants to let into the country.
Each immigrant profile includes a diverse set of attributes like whether they have made prior trips to the U.S., country of origin, profession, education level, and gender. An example of what respondents might see is included below in figure 4. Importantly, each attribute in the profile has a set of distinct levels and each profile is randomly generated using these possible attribute values. In all, each respondent completed this exercise with 5 different pairs of immigrant profiles.
Now, we want to consider immigration and who is permitted to come to the United States to live. For the next few minutes, we are going to ask you to act as if you were an immigration official.
We will provide you with several pieces of information about people who might apply to move to the United States. For each pair of people, please indicate which of the two immigrants you would personally prefer to see admitted to the United States. This exercise is purely hypothetical. Please remember that the United States receives many more applications for admission than it can accept. Even if you aren’t entirely sure, please indicate which of the two you prefer.
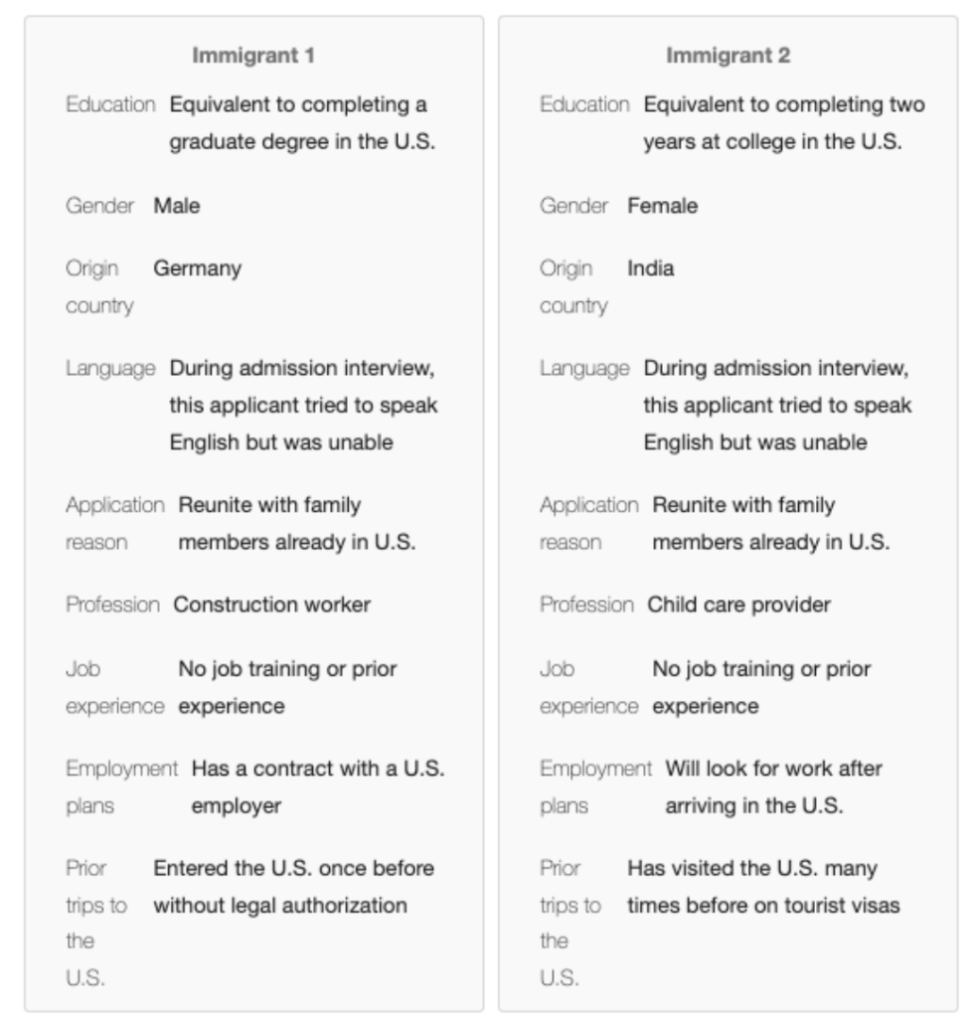
Conjoints are a useful tool in survey research that has grown in popularity over the last two decades. Conjoint experiments provide respondents with a realistic scenario that mimics decisions that respondents make in their day-to-day lives. With enough responses, conjoint experiments allow researchers to estimate treatment effects for a wide range of variables at once and measure the relative importance of different attributes.³
In the original paper, Hainmueller and Hopkins find that Americans tend to prefer immigrants with higher levels of education, who speak English well, and who have certain professions like construction workers, nurses, and research scientists. Perhaps surprisingly, they also find that there are few significant differences in immigrant preferences based on respondent age, gender, or partisanship; in the author’s words, there is a “hidden consensus” on attitudes towards immigrants.
In our study, we analyzed the results of the conjoint experiment for each of the three samples with all respondents included, and then with only the respondents who passed our quality checks. Because results for all three panels were consistent, we show the combined results here for clarity.
We find that among all respondents, only one of the 34 AMCEs has an opposite sign compared to the original paper, and that among good respondents all 34 AMCEs have the same sign. In experiment three, effect sizes among both all respondents and the subset of good respondents was somewhat lower than the effects reported in the original paper. Removing poor-quality respondents shrank the gap between our results and the published results by around 10%. Given that the original experiment was published nearly a decade ago and that immigration continues to be a salient political issue, it is understandable that we find some difference in the effect size, but here again we see that the effects are larger among the good subset than among the set of all respondents.
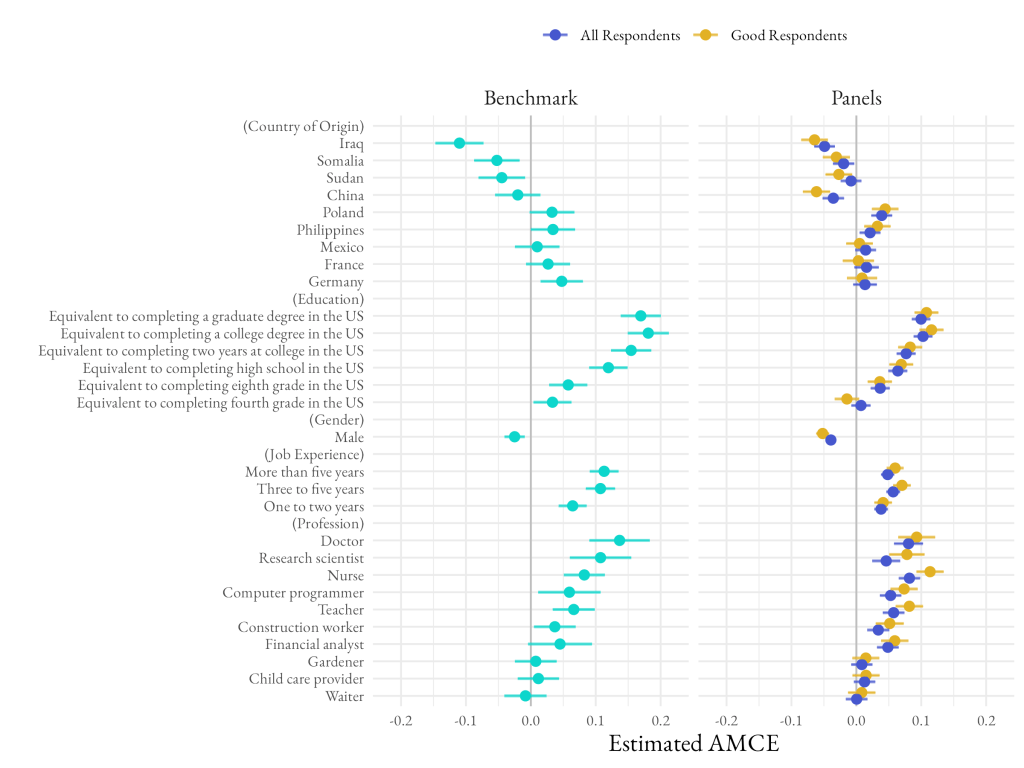
Conclusion
The sum of our results suggests two main conclusions. First, experimental results are absolutely replicable using non-probability samples from online panels. Even without any quality controls, we found directionally similar results across three different panels and three different survey experiments. Second, poor-quality responses do introduce noise to estimates of treatment effects. On average, removing duplicate, fraudulent, and inattentive respondents led to effect sizes around 30% larger.
Notes
¹ Throughout this article we have removed the names of each sample source. All three are large panels or exchanges commonly used throughout the industry.
² In total, nearly 1,800 cases were flagged by one of these quality checks, representing between 28% and 33% of the total sample from each panel.
³ Conjoint experiments are useful in a wide range of contexts. For our take on a novel use of conjoint experiments in pricing research, see our blog post here.
References
Aronow, P. M., Kalla, J., Orr, L., & Ternovski, J. (2020). Evidence of rising rates of inattentiveness on Lucid in 2020. SocArXiv, 49, 59-63.
Bargh, J. A., Chen, M., & Burrows, L. (1996). Automaticity of social behavior: Direct effects of trait construct and stereotype activation on action. Journal of personality and social psychology, 71(2), 230.
Berinsky, A. J., Margolis, M. F., & Sances, M. W. (2014). Separating the shirkers from the workers? Making sure respondents pay attention on self‐administered surveys. American journal of political science, 58(3), 739-753.
Buzbas, E. O., Devezer, B., & Baumgaertner, B. (2023). The logical structure of experiments lays the foundation for a theory of reproducibility. Royal Society Open Science, 10(3), 221042.
Clifford, S., Sheagley, G., & Piston, S. (2021). Increasing precision without altering treatment effects: Repeated measures designs in survey experiments. American Political Science Review, 115(3), 1048-1065.
Doyen, S., Klein, O., Pichon, C. L., & Cleeremans, A. (2012). Behavioral priming: it’s all in the mind, but whose mind?. PloS one, 7(1), e29081.
Hainmueller, J., & Hopkins, D. J. (2015). The hidden American immigration consensus: A conjoint analysis of attitudes toward immigrants. American journal of political science, 59(3), 529-548.
Ioannidis, J. P. (2005). Why most published research findings are false. PLoS medicine, 2(8), e124.
Jerit, J., & Barabas, J. (2023). Are Nonprobability Surveys Fit for Purpose?. Public Opinion Quarterly, 87(3), 816-840.
Maniadis, Z., Tufano, F., & List, J. A. (2017). To replicate or not to replicate? Exploring reproducibility in economics through the lens of a model and a pilot study.
Mercer, A., & Lau, A. (2023). Comparing two types of online survey samples. Pew Research. Available at: https://www.pewresearch.org/methods/2023/09/07/comparing-two-types-of-online-survey-samples/
Mercer, A., Kennedy, C., & Keeter, S. Online opt-in polls can produce misleading results, especially for young people and Hispanic adults. Pew Research. Available at: https://www.pewresearch.org/short-reads/2024/03/05/online-opt-in-polls-can-produce-misleading-results-especially-for-young-people-and-hispanic-adults/
Open Science Collaboration. (2015). Estimating the reproducibility of psychological science. Science, 349(6251), aac4716.
Piston, S. (2018). Class attitudes in America: Sympathy for the poor, resentment of the rich, and political implications. Cambridge University Press.Tversky, A., & Kahneman, D. (1981). The framing of decisions and the psychology of choice. science, 211(4481), 453-458.

Alexander Podkul, Ph.D., is Senior Director of the Research Science team at Morning Consult, where his research focuses on sampling, weighting, and methods for small area estimation. His extensive background of using quantitative research methods with public opinion survey data has been published in Harvard Data Science Review, The Oxford Handbook of Electoral Persuasion and more. Alexander earned his doctorate, master's degree and bachelor's degree from Georgetown University.

James Martherus, Ph.D. is a senior research scientist at Morning Consult, focusing on online sample quality, weighting effects, and advanced analytics. He earned both his doctorate and master's degree from Vanderbilt University and his bachelor's degree from Brigham Young University.